PRECISE-AI
Performance and Reliability Evaluation for Continuous Modifications and Useability of Artificial Intelligence
The Big Question
What if AI models in health care autocorrected to maintain peak clinical performance?
The Problem
Artificial Intelligence (AI) is becoming an increasingly important tool used to help support clinical decision making. Since 2018, the number of available AI-enabled medical devices in the U.S. has increased by tenfold and will likely continue growing at similar rates in the future. However, research suggests that the accuracy of Machine Learning (ML) models may degrade over time due to changes in input data – such as changes in clinical operations, data acquisition, patient population, or even IT infrastructure. The accuracy of AI models in health care is paramount, as an inaccurate output could have dire consequences for a patient’s health outcome and the efficacy of our health system.
The Current State
Despite these issues, no current clinical AI models receive regular testing during clinical use to ensure that the accuracy of output is maintained. There are also no requirements to update AI models whose performance have degraded, in part because of a lack of technical solutions. Today, the main method of detecting degradation within AI models is clinical intuition on the part of physician using the technology. However, relying on clinical intuition can be unreliable and highly variable, meaning that AI model degradation may have already caused misdiagnosis before it is noticed.
The Challenge
To address these issues, the Performance and Reliability Evaluation for Continuous Modifications and Useability of Artificial Intelligence (PRECISE-AI) program aims to develop capabilities that can automatically detect and mitigate AI model degradation. These tools will monitor the performance of clinical AI models, identify if a degradation has occurred, and provide capabilities that can correct for performance degradations without the need for human oversight, thereby reducing the burden on individual operators. Importantly, this technology will also communicate clear and actionable information about the sources of degradation and allow users to better interpret model uncertainty, and thus help them use their software more effectively.
The Solution
PRECISE-AI aims to bring together machine learning experts, health information specialists, and clinicians to address five technical areas. TA 1 focuses on the automatic extraction and integration of data across different clinical use cases to establish a “ground truth” about each patient. TA 2 seeks to continuously monitor model performance, determine root causes of degradation, and suggest or make automatic corrections when needed. TA 3 aims to quantify uncertainty and improve clinical outcomes by finding novel ways of communicating model uncertainty and complementary measures to clinicians, developers, and other stakeholders. TA 4 will aggregate and share data across medical institutions and across performers to advance development of TA 1-3. TA 5 will confirm the progress made by all the TAs by performing independent verification and validation.
Why ARPA-H?
To succeed, PRECISE-AI will require interdisciplinary coordination between leaders across multiple fields, including artificial intelligence, health informatics, medical imaging, and much more. Given its broad mandate, ARPA-H is uniquely positioned to facilitate such cooperation, while also taking on the inherent risk associated with such an ambitious and transformative agenda.
Program Manager
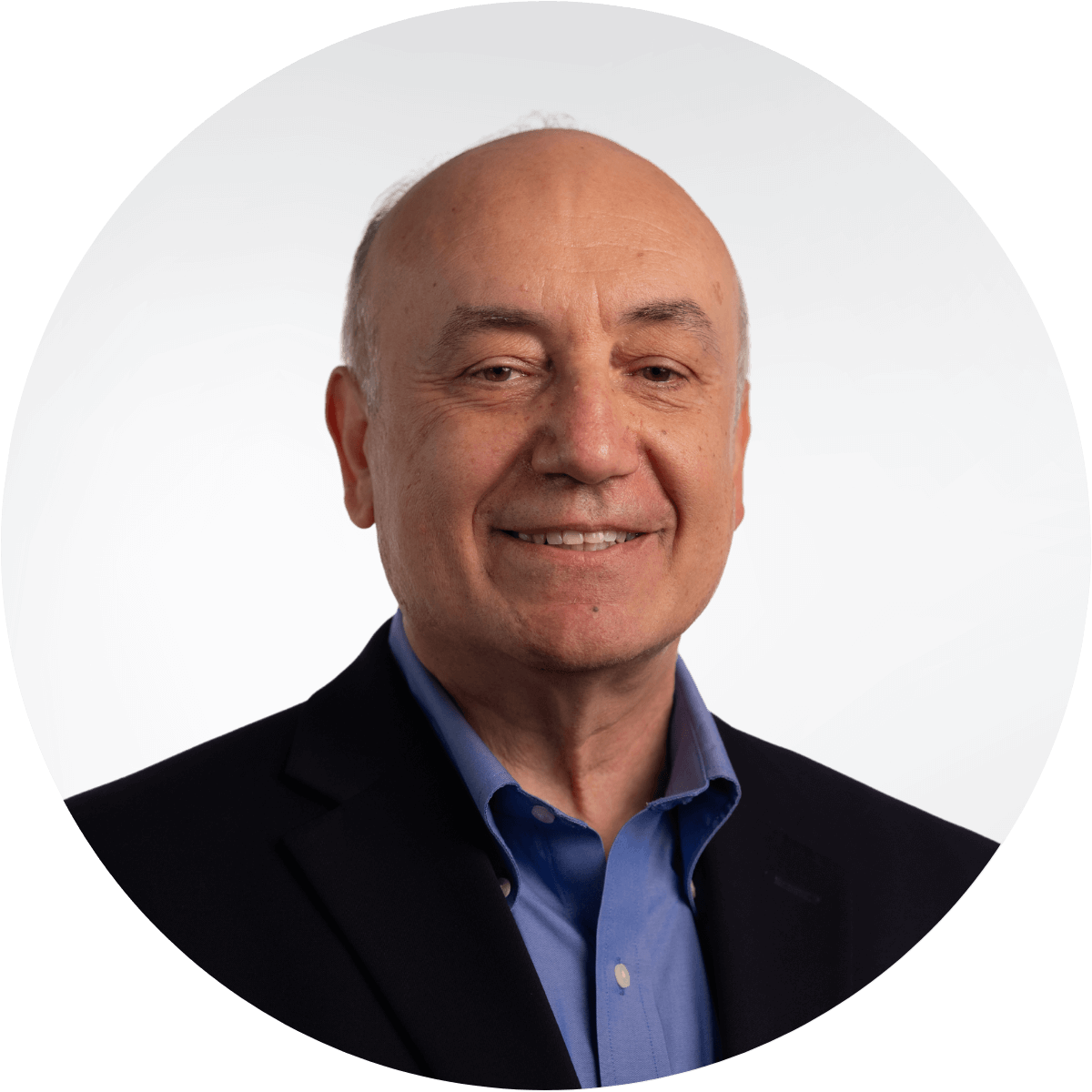
Berkman Sahiner, Ph.D.
Solicitation
Solicitation is closed.